The use of artificial intelligence (AI) in healthcare has a substantial environmental footprint but it could still have net carbon benefits, particularly in radiology, researchers reported.
Data storage and computational efforts tied to AI are increasing contributors to greenhouse gas emissions, particularly in radiology, which is a leader in healthcare AI, Kate Hanneman, MD, MPH, of the University of Toronto, and colleagues wrote in a review in Radiology.
Yet AI can also improve environmental sustainability in medical imaging by reducing MRI scan times, improving the scheduling efficiency of scanners, and optimizing decision-support tools to reduce low-value imaging, Hanneman and co-authors noted.
“AI applications potentially generate large amounts of greenhouse gases, mostly related to their energy use. But on the flip side, there is the potential to improve environmental sustainability,” Hanneman told MedPage Today. “This is the start of a conversation.”
AI contributes to greenhouse gas emissions in two ways, Hanneman observed. First, the process of training, validating, and deploying AI models requires significant computational power, which means more energy use. Second, AI computing requires significant data storage, which increases energy demands.
Computer and server rooms and data warehouses “require a lot of energy to power and to cool,” Hanneman said.
This certainly applies to radiology, Hanneman said, though there is a lack of data on the specialty’s specific impact, such as energy estimates for computing and storing of data specific to radiology tools.
“We’re making estimates and inferences based on non-radiology data, but there’s a huge need for further research in this area to really understand … what is the actual energy use? What are the actual associated greenhouse gas emissions?” Hanneman said. “There’s a bit of a gap there, so we need more data.”
At the same time, AI may also be able to create solutions for radiology that could produce net environmental benefits. For instance, CT and MRI scanners consume a substantial amount of energy even when idle, which AI tools could address with automated system shutdowns.
Also, since scan duration has a proportional relationship to energy consumption, AI-based applications could help reduce MRI scan times, and thus energy expenditure, the researchers wrote.
Both of these applications could reduce energy use and greenhouse gas emissions while lowering costs for radiology practices, they noted.
Radiology departments will need to optimize energy use for AI models by adopting new approaches to implementing the technology, including use of low-power processing units, the authors suggested.
There’s also the problem of the “proliferation of numerous AI models developed independently by different groups to address similar questions,” they wrote, such as similar models to identify pulmonary emboli on CT scans. “Efforts targeted at fostering collaboration and resource sharing would decrease overall greenhouse gas emissions associated with AI development while also enhancing the external validity of the resulting models,” they wrote, calling for multi-institution collaboration.
Additionally, healthcare companies should “carefully evaluate their data storage choices to enhance environmental sustainability,” as data centers in cooler climates or where renewable energy sources are available would be more beneficial.
“Departments that manage their cloud storage can take immediate action by choosing a sustainable partner,” Hanneman said in a statement.
While gaps in knowledge as to radiology’s AI environmental footprint remain, Hanneman said, the goal is to open “a chapter in radiology in terms of thinking about the implications of AI, both the positives and the negatives.”
Disclosures
The authors reported financial relationships with several companies and grant organizations.
Primary Source
Radiology
Source Reference: Doo FX, et al “Environmental sustainability and AI in radiology: A double-edged sword” Radiology 2024; DOI:10.1148/radiol.232030.
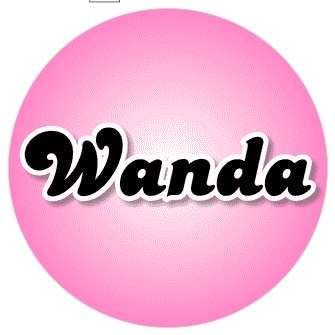
Wanda Parisien is a computing expert who navigates the vast landscape of hardware and software. With a focus on computer technology, software development, and industry trends, Wanda delivers informative content, tutorials, and analyses to keep readers updated on the latest in the world of computing.