In a recent paper titled “Extended SLIC superpixels algorithm for applications to non-imagery geospatial rasters” published in the International Journal of Applied Earth Observation and Geoinformation, researchers from Adam Mickiewicz University and University of Cincinnati presented an enhanced version of the widely-used SLIC algorithm for image segmentation, known as extended SLIC. This algorithm is designed specifically for multidimensional spatial raster datasets and offers improved regionalization capabilities.
The study demonstrates that the extended SLIC algorithm, which utilizes “native” data distance, can achieve better segmentation and regionalization results. The algorithm was tested on three datasets with varying dimensions and compressibility: land cover fractions, climatic time series, and elevation data.
In the first testing example, the objective was to create regions with homogeneous land cover. The extended SLIC algorithm outperformed the original SLIC algorithm in terms of segmentation accuracy, despite the high dimensionality of the data.
In the second example, the authors applied the algorithm to climatic time series raster data with 24 variables, aiming to create regions with similar temperature and precipitation variability. Here, the extended SLIC algorithm showed a slight advantage over the original SLIC algorithm. Finally, the authors used a set of topographic features to detect dunes in Algeria as the third example, which involved the least compressible data among the three examples. In this case, the extended SLIC algorithm demonstrated a significant advantage over the original SLIC algorithm.
The results indicate that the benefit of the extended SLIC algorithm is inversely proportional to the compressibility of the data, specifically up to three dimensions. The raster of climatic time series, being the most compressible data, yielded mixed results, with five out of ten metrics favoring the extended SLIC and four favoring the original SLIC. However, the topographic features dataset, with the least compressible data, showed a significant advantage for the extended SLIC algorithm.
This research carries significant implications for spatial regionalization, image segmentation, and data analysis in fields like remote sensing, where complex, high-dimensional datasets are common. It emphasizes the necessity for algorithms that can efficiently analyze such data and provide superior outcomes compared to existing methods. Overall, this study contributes to the ongoing efforts to enhance image segmentation and data analysis techniques for complex multidimensional spatial datasets.
More information:
Jakub Nowosad et al, Extended SLIC superpixels algorithm for applications to non-imagery geospatial rasters, International Journal of Applied Earth Observation and Geoinformation (2022). DOI: 10.1016/j.jag.2022.102935
Provided by
Adam Mickiewicz University
Citation:
Spatial regionalization algorithm shows promising results in complex datasets (2023, July 28)
retrieved 29 July 2023
from https://phys.org/news/2023-07-spatial-regionalization-algorithm-results-complex.html
This document is subject to copyright. Apart from any fair dealing for the purpose of private study or research, no
part may be reproduced without the written permission. The content is provided for information purposes only.
Denial of responsibility! TechCodex is an automatic aggregator of the all world’s media. In each content, the hyperlink to the primary source is specified. All trademarks belong to their rightful owners, and all materials to their authors. For any complaint, please reach us at – [email protected]. We will take necessary action within 24 hours.
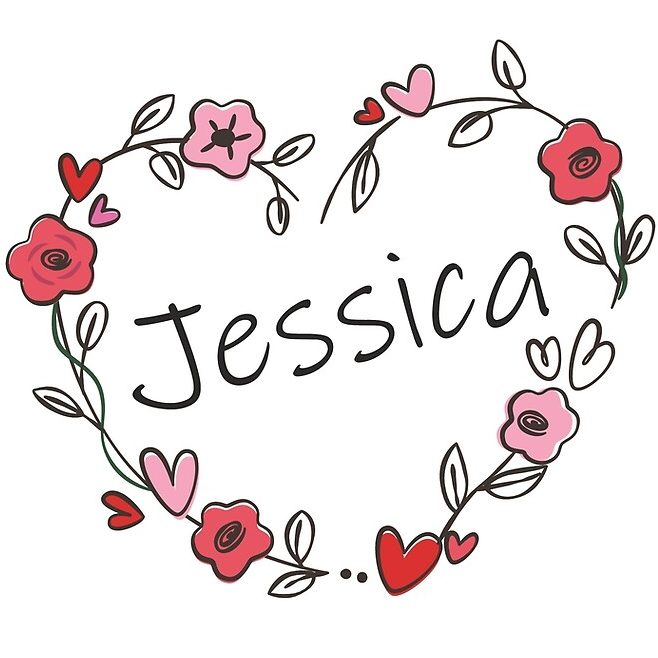
Jessica Irvine is a tech enthusiast specializing in gadgets. From smart home devices to cutting-edge electronics, Jessica explores the world of consumer tech, offering readers comprehensive reviews, hands-on experiences, and expert insights into the coolest and most innovative gadgets on the market.