A staff member at London’s Royal Academy studies the Jackson Pollock painting “Blue Poles” in … [+]
Can a machine paint like Jackson Pollock? A team out of Harvard says yes. The scientists combined artificial intelligence and physics to invent a 3D-printing technique that replicates the artist’s career-defining paint-flinging approach. Their ultimate goal, however, isn’t to design a Pollock driven by computer algorithms, but to print complex shapes more quickly.
The method, which the researchers describe in the latest issue of Soft Matter, leverages the same rules of fluid dynamics Pollock relied on when he famously put canvases on the floor and dripped, drizzled and poured paint on them from above.
The American abstract-expressionist artist counted on paint’s instability—the way it buckled, folded and coiled as gravity pulled it down to the surface—to create fascinating, intricate patterns below. By moving his hands and body, Pollock intuitively leaned on laws of physics to control the flow of paint, resulting in works which, in the Museum of Modern Art’s words, “present less a picture than a record of the fluid properties of paint itself.”
Current 3D- and 4D-printing methods avoid the dynamic instability of the liquid stream by placing a nozzle just millimeters from a surface and sending it along a specified print path from point A to point B. That works fine, but because the nozzle has to deposit ink or other printing materials at every point along its trajectory, the process can be slow. What’s more, the proximity of the nozzle to the surface hinders the kind of creative latitude afforded by welcoming natural fluid instability rather than avoiding it.
“Pollock’s approach of throwing paint from a height meant that even if his hand was moving in a specific trajectory, the paint didn’t follow that trajectory because of the acceleration gained from gravity,” Gaurav Chaudhary, a former postdoctoral fellow at Harvard’s School of Engineering and Applied Sciences and first author of the research paper, said in an interview. “A small motion could result in a large splatter of paint. Using this technique, you can print larger lengths than you can move because you gain this free acceleration from gravity.”
That’s what most stands out to Tyler Ley, an engineering professor Oklahoma State University. “The most exciting breakthrough I see is that current 3D-printer nozzles have a fixed diameter,” Ley, who is not involved with the Harvard research, said in an email. “This approach will allow variable diameter widths by varying the height and the rate of deposition.”
A cursive “Cambridge” 3D-printed using deep reinforcement learning, which lets software agents learn … [+]
Chaudhary worked with a team led by L. Mahadevan, a Harvard professor of applied mathematics, engineering, physics and organismic evolutionary biology. More than 20 years ago, Mahadevan offered a physical explanation of fluid coiling.
In the research paper published in Soft Matter, the team members demonstrate how they trained AI to manipulate a nozzle to mimic Pollock paintings and write in cursive 2.5 times faster than traditional 3D-printing can. They mostly used viscous silicone oil filaments in their experiments. At one point, though, they decorated a cookie by dropping chocolate syrup from above to show how a deep reinforcement learning agent can be deployed to print on edible surfaces.
Chaudhary said the new approach could make it easier to adapt current printing practices to complex, non-planar substrates such as spheres.
A welcome ‘dash of unpredictability’
New Zealand engineer Michelle Dickinson, who’s not connected to the research, expressed enthusiasm about the creative potential of printing the Pollock way.
“It brings a dash of unpredictability to a field that has previously strived to be accurate and precise,” Dickinson, founder of Nanogirl Labs, which aims to inspire and educate through STEM, said in an email. “The great thing about this method is its ability to add the impression of artistic flair to a relatively predictable printing method and aesthetically produce works that look more human-made than machine-made.”
To replicate the dynamics of fluid coils, the Harvard researchers combined fluid simulations and deep reinforcement learning, which allows software to get better at performing a task by learning from its mistakes. They say their approach could be expanded to include more complex fluids, such as liquid polymers and pastes, and to stack multiple layers.
Dickinson said she could envision the technique advancing tissue engineering. It “could be used to print collagen scaffolds out of liquid biopolymers which mimic the more natural structures found in the body,” she said.
For now, the research into “printing at a distance” continues, as does the world’s fascination with Pollock’s work.
Denial of responsibility! TechCodex is an automatic aggregator of the all world’s media. In each content, the hyperlink to the primary source is specified. All trademarks belong to their rightful owners, and all materials to their authors. For any complaint, please reach us at – [email protected]. We will take necessary action within 24 hours.
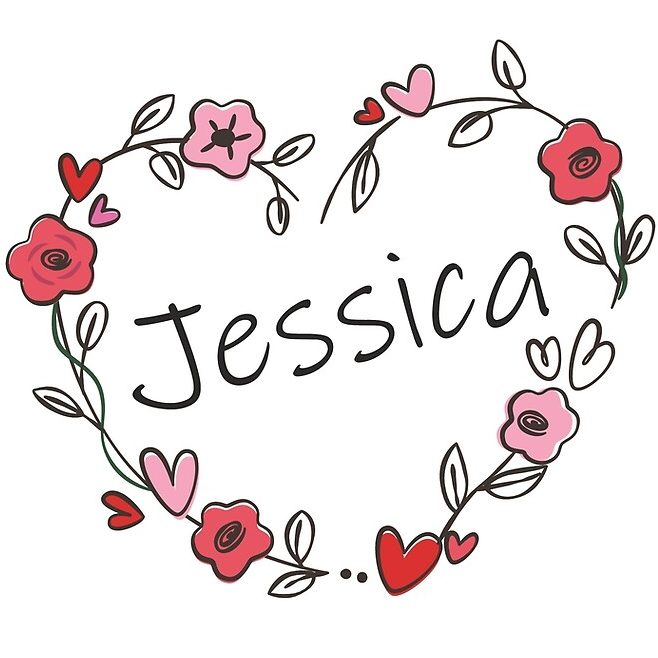
Jessica Irvine is a tech enthusiast specializing in gadgets. From smart home devices to cutting-edge electronics, Jessica explores the world of consumer tech, offering readers comprehensive reviews, hands-on experiences, and expert insights into the coolest and most innovative gadgets on the market.