Researchers have long been fascinated by metal-organic frameworks (MOFs) due to their diverse range of applications, including gas absorption, water harvesting, energy storage, and desalination. However, selecting the most effective MOFs for specific tasks quickly and cost-effectively has posed a challenge. This is where MOFormer, a machine learning model, comes in. Unlike existing models that rely on 3D atomic structures, MOFormer can achieve higher accuracy in prediction tasks without explicitly depending on these structures.
“We realized that relying on the costly 3D structures of MOFs was unnecessary. To overcome this, we used MOFids to make accurate predictions,” explained Yuyang Wang, a Ph.D. student in the research group of Professor of Mechanical Engineering Amir Barati Farimani.
The research was recently published in The Journal of the American Chemical Society.
A MOFid is a text string representation of the building blocks of MOFs, which include metal nodes, organic linkers, and topologies. These representations enable machine learning models to predict properties. However, due to the countless combinations of building blocks, finding optimal MOFs is complex. In MOFormer, researchers can quickly screen MOFs by creating new MOFids hypothetically.
“To train MOFormer, we utilized self-supervised learning (SSL), which combines structure-based and structure-agnostic approaches. SSL improves the performance of MOFormer in predicting properties by maximizing the mutual information between these approaches,” explained Rishikesh Magar, a Ph.D. student in Barati’s lab.
“This allows researchers to efficiently build MOFs. For example, if there are one million hypothetical MOF structures that may be suitable for water harvesting, MOFormer can predict the most desirable properties, narrowing down the options. From there, researchers may only need to test 100 variations in the lab,” illustrated Zhonglin Cao, another Ph.D. student in Barati’s group.
Compared to other structure-agnostic methods, MOFormer achieves 35-48% higher accuracy in various gas absorption prediction tasks and 21.4% higher accuracy in band gap prediction.
“We believe MOFormer will accelerate the exploration of MOFs. It can serve as a valuable tool for investigating the vast chemical space of hypothetical MOFs,” stated Farmini.
More information:
Zhonglin Cao et al, MOFormer: Self-Supervised Transformer Model for Metal–Organic Framework Property Prediction, Journal of the American Chemical Society (2023). DOI: 10.1021/jacs.2c11420
Provided by
Carnegie Mellon University Mechanical Engineering
Citation:
More metal-organic frameworks, fewer problems: A self-supervised transformer model for property prediction (2023, July 31)
retrieved 1 August 2023
from https://phys.org/news/2023-07-metal-organic-frameworks-problems-self-supervised-property.html
This document is subject to copyright. Apart from any fair dealing for the purpose of private study or research, no
part may be reproduced without the written permission. The content is provided for information purposes only.
Denial of responsibility! TechCodex is an automatic aggregator of the all world’s media. In each content, the hyperlink to the primary source is specified. All trademarks belong to their rightful owners, and all materials to their authors. For any complaint, please reach us at – [email protected]. We will take necessary action within 24 hours.
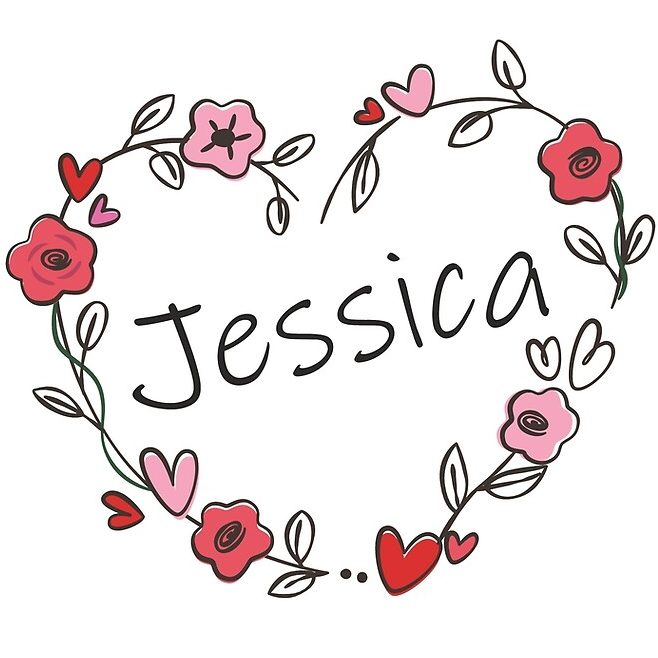
Jessica Irvine is a tech enthusiast specializing in gadgets. From smart home devices to cutting-edge electronics, Jessica explores the world of consumer tech, offering readers comprehensive reviews, hands-on experiences, and expert insights into the coolest and most innovative gadgets on the market.