The aim of this study was to perform an external validation, in real clinical practice, of the diagnostic capability of an AI algorithm with respect to the reference radiologist for chest X-rays, as well as to detect possible diagnoses for which the algorithm had not been trained. Thus, the overall accuracy of the algorithm was 0.95 (95% CI 0.92–0.98), the sensitivity was 0.48 (95% CI 0.30–0.66) and the specificity was 0.98 (95% CI 0.97–0.99). The results obtained have further highlighted, as indicated by different expert groups26,28,29, the need for external validations of AI algorithms in a real clinical context in order to establish the necessary measures and adaptations to ensure safety and effectiveness in any environment. Therefore, in the context of the model developed, it is important to understand and interpret what each of the results obtained indicate.
High accuracy values were observed in most cases (ranging between 0.7–1). The accuracy is represented by the proportion of correctly classified results among the total number of cases examined. This value was high since, both for each condition and for the groups of conditions, the capacity to detect true negatives was good, taking into account that most of the images analysed were found to have no abnormalities (51.8%). Working with an AI algorithm that quickly determines that there is no abnormality can function as a triage tool, streamlining the diagnostic process, allowing the professional to focus on other tests, reduce waiting lists, reduce waiting times for diagnoses and even reduce expenses in secondary tests.
With sensitivity referring to the ability to detect an abnormality when there really is one, high sensitivity values were shown when detecting anatomical findings or abnormalities such as sternal cables, enlarged heart, abnormal ribs, spinal implants, cardiac valve, or interstitial markings. On the other hand, low sensitivity values were observed for most conditions, indicating that the algorithm had limited ability to detect certain conditions like those in the mediastinum, vessels, or bones. These findings align with the results of a study that performed an external validation of a similar algorithm in an emergency department35. Additionally, the algorithm exhibited low sensitivity in detecting pulmonary emphysema, linear atelectasis, and hilar prominence, which are prevalent conditions in the primary care setting31.
Low sensitivity was also observed when detecting nodules, with the algorithm finding more nodules than the reference radiologist, in most cases confusing them with areolae in the breast tissue. Although it is important to be able to detect any warning signs and that the professional is in charge of making the clinical judgement and determining the need for complementary tests, it is possible that this external validation has detected a possible gender bias in the training of the algorithm. When it comes to chest imaging, it’s important to distinguish between the physiological aspects of breast tissue and any potential changes it may undergo during various life stages, as opposed to signs of conditions or abnormalities36. Other studies have also detected a high false positive value in the detection of nodules due to other causes such as fat, pleura or interstitial lung disease37.
Finally, specificity being the ability to correctly identify images in which there are no radiological abnormalities, the results showed high values for all condition groupings, since the algorithm was able to detect images with no abnormalities.
Following the authors’ desire to contribute to the improvement of the AI model, some radiologists’ findings were identified that were overlooked during the algorithm’s training, especially related to bronchial conditions, including chronic bronchopathy, bronchiectasis, and bronchial wall thickening. Additionally, the algorithm missed common chronic conditions often seen in primary care, including chronic pulmonary abnormalities, COPD, and fibrocystic abnormalities. Furthermore, it was noted that certain condition names within the AI algorithm should be adjusted to align with names used in the radiology field. Interstitial markings could be changed to interstitial abnormality, consolidation to condensation, aortic sclerosis to valvular sclerosis, and abnormal rib to rib fracture.
Once the main variables that characterise the algorithm’s capacity were discussed, the results obtained differ from the majority of published studies, since most of them obtained a higher algorithm capacity. However, it should be noted that most of these are internal validations and not tested in real clinical practice settings38,39,40.
A study in Korea performed an internal and external validation of an AI algorithm capable of detecting the 10 most prevalent chest X-ray abnormalities and was able to demonstrate the difference in sensitivity and specificity values. The internal validation obtained sensitivity and specificity values between 0.87–0.94 and 0.81–0.98, respectively. On the other hand, the external validation obtained sensitivity and specificity values between 0.61–1.00 and 0.71–0.98, respectively41. This difference can also be seen in a study in Michigan, where internal and external validation of an AI algorithm capable of detecting the most common chest X-ray abnormalities was performed42, and in a study at the Seoul University School of Medicine, where an algorithm for lung cancer detection in population screening was validated43.
Therefore, the results obtained from the external validation show the need to increase the sensitivity of the algorithm for most conditions. Considering that AI should serve as a diagnostic support tool and the ultimate responsibility for medical decisions rests with the practitioner, it is ideal for the algorithm to flag potential abnormalities for the practitioner to review and confirm. This ensures the highest diagnostic effectiveness. Recent studies have shown that the use of an AI algorithm to support the practitioner significantly improves diagnostic sensitivity and specificity and reduces image reading time20,44.
Enhanced sensitivity could help address the shortage of specialised radiologists globally, especially in Central Catalonia’s primary care setting, where this validation was conducted45,46. More and more, general practitioners are tasked with interpreting X-rays. In this context, the advancement of these tools can be a valuable asset in the diagnostic process.
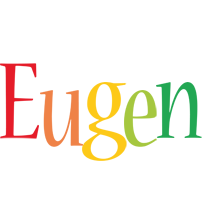
Eugen Boglaru is an AI aficionado covering the fascinating and rapidly advancing field of Artificial Intelligence. From machine learning breakthroughs to ethical considerations, Eugen provides readers with a deep dive into the world of AI, demystifying complex concepts and exploring the transformative impact of intelligent technologies.