Anyone following the discourse on governance and policy-making regarding artificial intelligence (AI) will likely encounter some form of discussion on AI “standards.” These discussions on standards are seen across different governance contexts, including official government instruments that have proposed framing standards, such as the USA’s Executive Order on AI (Executive Order on AI), and international standard-setting bodies like ISO that have developed AI-related standards. But why is there a need for these standards? And who decides what these AI “standards” should look like?
A wide range of standards are present across industries today – be it food, health, finance, or technology. Industry standards can serve different purposes, including providing a consistent way of evaluating and measuring the quality of products, promoting interoperability, and consumer safety and protection. There have been multiple instances of AI products and services causing harm to users when deployed in the market. From being inadvertently trained on datasets containing child sexual abuse material to producing copyrighted material in user outputs to incorrectly identifying individuals accused of committing crimes – AI products and services can have unintended problems, with consequences impacting end users. Thus, there is a need to develop “standards” – which can include technical and management measures – to ensure that AI companies follow best practices consistently while developing and deploying AI products and services.
Existing sources of standards for industry, government, and international bodies
Previous online technology services like social media platforms and e-commerce stores largely escaped regulation during their initial development and mass user adoption stages. However, AI, specifically generative AI, has become the target of regulation much earlier. For example, the US government’s Executive Order on the Safe, Secure, and Trustworthy Development and Use of Artificial Intelligence requires “consensus industry standards for developing and deploying safe, secure, and trustworthy AI systems.” The European Union’s AI Act also references standards.
But where will these standards come from? One source is international standard-setting bodies. The AI Standards Hub contains a database of almost 300 AI standards, classified on the basis of multiple factors. One factor is the issuing body; standards can be issued by both domestic bodies, such as the American National Standards Institute (ANSI) and the National Institute of Standards and Technology (NIST), and international ones, such as the ISO and the International Telecommunications Union (ITU). There are sector-specific AI standards focused on areas like healthcare and the environment. There are also AI standards for different technological applications, such as facial recognition, natural language processing, and robotics.
If there are already ~300 published AI standards, isn’t the problem already solved? Not exactly. It is difficult to ascertain the status of the adoption of these standards by the AI industry. Many factors impact the adoption of international industry standards, including the incorporation of standards into law or regulations, market competition, and the need for companies to establish credibility. Given how nascent the technology is, one could argue that AI standards merely serve as guidance for companies.
The lack of an existing legal mandate on the adoption of AI standards is one primary reason why government and legislative instruments such as the Executive Order on AI and the European Union’s AI Act include measures to facilitate the creation of industry standards. Both national and international standard-setting organizations often assist governments in creating standards that are later enforced through government actions. For example, the Executive Order on AI has tasked the NIST, which previously released a voluntary AI Risk Management Framework, to produce guidelines and resources to facilitate AI standards in several new areas.
But even without legal mandates, the AI industry is responding to calls for standardization through its own de facto AI standards in the form of industry best practices.
The emergence of de facto AI standards based on industry best practices
Many countries have laws that protect social media platforms against legal liability arising from content posted by their users, subject to limited exceptions (such as child pornography). The scope of these intermediary liability protections varies across countries. Nevertheless, even before most countries enacted laws governing the contours of safe harbor protection, social media platforms developed internal policies such as community standards and user complaint mechanisms.
A practice started by one company would often turn into a common industry practice. For example, Twitter first introduced account verification marks, which were later adopted by Facebook and Instagram. While social media companies initially adopted many of these measures voluntarily, some countries have mandated them through law. For example, the EU’s Digital Services Act, which came into force in February 2024, makes it mandatory for online platforms to have internal complaint-handling mechanisms; India also updated its laws in 2021 to require online platforms to implement a grievance redressal mechanism for users, among other obligations. Thus, measures that started as voluntary industry practices became de facto standards, and in some cases, even legal standards. Something similar also seems to be happening with AI, specifically generative AI.
Many generative AI services have started developing their version of content-moderation policies, generally referred to as an ‘acceptable use policy’ or ‘usage policy.’ These policies are intended to assuage concerns arising from users asking chatbots to generate harmful content. For example, OpenAI’s usage policy specifies that users should not use its services to “promote suicide or self-harm” or “develop or use weapons.” Concerns about copyright infringement, deepfakes, and misinformation have also led companies like Meta and YouTube to announce content-labelling requirements allowing users to identify AI-generated content. In response to the growing number of copyright lawsuits, OpenAI also introduced an opt-out form to enable artists to exclude their material from being used by OpenAI for training purposes.
Importantly, content-related measures – whether to prevent copyright infringement or user harm – are just one category of industry measures. Companies also take measures related to other areas, such as safety (such as through red-teaming practices), access (e.g., having open access models such as Meta’sLlama 2 or closed access models like the recently announced Mistral Large), and transparency (e.g., publishing model cards).
Standards are also under development through industry collaborations. For example, one major issue often discussed for generative AI and synthetic media is content provenance, i.e., ascertaining the source of any content and its history in terms of modifications made to it. Content provenance and labeling have become highly discussed solutions to the rising concern around the proliferation of deepfakes.
The Coalition for Content Provenance and Authenticity (C2PA) developed a technical standard called “Content Credentials” to introduce transparency in the provenance of digital content. C2PA is an industry initiative initially led by Adobe, Arm, Intel, Microsoft, and Truepic; Google is the latest company to join the initiative. Recently, BBC implemented the Content Credentials standard to provide users with more information about the origin of an image or video and how BBC has verified its authenticity.
Similarly, the International Press Telecommunications Council developed the IPTC Photo Metadata Standard; Midjourney and Shutterstock AI agreed to adopt this standard. Another recent example is the AI Elections Accord, a voluntary set of commitments agreed to by various companies to counter deceptive election content. These standards are also a good example of how the industry comes up with voluntary self-regulatory measures in anticipation of avoiding government measures that may not be preferable.
These industry practices are influencing legal and regulatory efforts as well. The White House AI Executive Order includes references to content provenance, labeling, and red-teaming, all of which are practices/terms popularized by the industry. In Recital 89, the EU AI Act mentions how developers of free and open-source tools should adopt documentation practices such as model cards –another voluntary measure popularized in the industry over the last couple of years.
Inconsistency in de facto industry standards
However, unlike proper “standards,” which would introduce consistency in industry practices through the backing of force of law (such as for sectors like food, environment, or finance), industry practices in AI are not followed consistently and are subject to the discretion of companies. An example of this is how model cards vary across organizations.
A model card is like a nutrition label for AI models. It discloses various information points about the model, including the training methodology, safety measures, etc. However, companies often pick and choose the information they wish to disclose in their model cards. While Meta disclosed the pre-training data in the model card of the first version of LLaMA, it has yet to do so for LlaMa 2 despite claiming that it is an open-access model.
Companies’ lack of consistent disclosures in open-source models is a concern for governments. For example, the National Telecommunications and Information Administration (NTIA), in a request for public input on dual-use foundation models with widely available model weights, noted how “openness” or “wide availability” of model weights are terms without a clear definition. Similarly, experts have concerns about the lack of clarity on the definition of models under a “free and open source license” in the EU AI Act, which exempts such models from many obligations of the law.
Another example is inconsistency in the removal of fake election-related images. A March 2024 report by the Center for Countering Digital Hate (CCDH) found that despite having official policies against election-related misinformation, many prominent AI-image generators, including ChatGPT Plus and Midjourney, allowed fake election-related images to be created. OpenAI announced in January 2024 that DALL-E contained safeguards to prevent generating images of real political candidates. The Associated Press found that Midjourney implemented similar measures against generating fake images of Joe Biden and Donald Trump a few days after the release of the CCDH report, which found that Midjourney performed the worst out of all tested tools.
These inconsistencies highlight the need for mandatory standards implemented through the force of law. Having standards designed either by international or domestic standard-setting bodies and then implemented domestically through government agencies or legislation would ideally introduce consistent requirements to be followed by AI companies.
How AI auditing organizations are emerging as an implementation solution for AI standards
While governments decide how to implement standards, another industry solution has emerged: AI auditing and consultancy organizations. In addition to helping organizations comply with existing/proposed legal frameworks on AI (such as the forthcoming EU AI Act or the New York legislation on the use of AI in employment), these consultancies also provide solutions to help organizations adopt AI more responsibly. For example, Credo.AI offers a “Generative AI Guardrails” solution that helps organizations manage potential risks from the adoption of generative AI, and BABL AI offers “Responsible AI Implementation Guidance.”
Some of these organizations also offer certifications based on their own assessment parameters. For example, the Responsible AI Institute offers a certification mark to organizations based on its own certification scheme. Some of these organizations have formed the International Association for Algorithmic Auditors, which seeks to act as an industry body for algorithmic auditing professionals and “lay the foundation for algorithmic auditing standards.”
While AI auditing may be driven by commercial demand, having an auditing ecosystem in place before any regulatory measures are introduced could help better implement such regulatory measures, supplementing the government’s capacity to implement new laws and policies. One way to increase the accountability and credibility of AI auditing consultancy firms could be to have a government-run accreditation scheme for such organizations.
Where do we go from here?
Different stakeholders are undertaking various efforts to better define AI standards. For example, in its recently released report on AI Accountability Policy, the National Telecommunications and Information Administration (NTIA) has highlighted how various stakeholders have highlighted the need for standards in different areas of AI accountability, such as auditing of AI systems and ascertaining liability for AI-based decision making. The Open Source Initiative (OSI) is a not-for-profit organization that advocates for open-source software. OSI recently proposed a draft definition of “Open Source AI” for public comments and is expected to finalize the definition over the next two months.
However, the task of framing standards for any sector is complicated. It is difficult to come up with one-size-fits-all standards that can then be applied consistently. Broad standards may be less effective, and narrow, strict standards may stifle innovation. As highlighted by Hadrien Pouget and Ranj Zuhdi at the Carnegie Endowment for International Peace, standards under the EU AI Act and existing AI-related standards by international bodies like IEEE or ISO do not provide concrete requirements as compared to standards from other fields.
As governments, regulatory agencies, and public-sector standard-setting bodies work toward enforceable AI standards, the industry is busy establishing its own set of best practices. These efforts are unlikely to be sufficient to establish industry-wide standards, but they can serve as an important source for governments and regulators to inform enforceable AI standards. Government intervention with regulatory backing will ensure that AI standards are enforceable and ultimately achieve the policy objective of making AI safer.
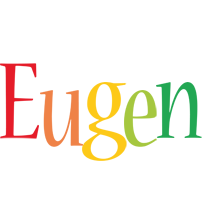
Eugen Boglaru is an AI aficionado covering the fascinating and rapidly advancing field of Artificial Intelligence. From machine learning breakthroughs to ethical considerations, Eugen provides readers with a deep dive into the world of AI, demystifying complex concepts and exploring the transformative impact of intelligent technologies.