Former Meta researchers who developed an AI language model for biology have raised $40 million to build new biological LLMs that can help develop everything from cancer-fighting programmable cells to organisms that clean up toxic waste.
Getty
Former Meta researchers who developed an AI language model for biology have launched a new startup and raised at least $40 million, Forbes has learned.
EvolutionaryScale is led by Alexander Rives, who ran Meta AI’s protein-folding team until the tech giant shuttered the project in April. The founding staff of eight all come from the same unit, where they created a transformers-based model in the vein of OpenAI’s GPT-4 or Google’s Bard, but trained on data about protein molecules in order to predict the structures of other unknown proteins. They used it to create a database that today contains 700 million possible 3D structures– key puzzle pieces for developing drugs that could cure diseases, microorganisms that could clean up pollution or alternative ways to manufacture industrial chemicals.
By June, the startup was pitching venture capitalists for seed financing to advance its research efforts by dramatically scaling up the size of its AI model, per a pitch document obtained by Forbes. Lux Capital led the roughly $40 million round, three people with knowledge of the deal said. One source said that the financing valued EvolutionaryScale at $200 million and that prominent AI investors Nat Friedman and Daniel Gross, participated in it.
Rives declined to comment, while Lux, Friedman and Gross did not respond to requests for comment.
Proteins are large molecules formed from folded chains of amino acids, and are the building blocks of bacteria, microbes and human cells. Their specific functions are often dictated by their shapes, and that shape can change when it interacts with other chemicals or proteins in the body. Accordingly, specific parts of a protein are often the targets of drugs to treat disease. But predicting the shape of a protein is complicated, because it’s governed by complicated interactions between the thousands of atoms within it. (For example, curly hair is caused by the interaction of sulfur atoms contained within a particular amino acid.) Such predictions are crucial in allowing scientists to figure out protein functions in order to design a drug that will properly bind to its three dimensional structure. The ability to map out the structure of so many proteins, then, helps the scientists to narrow their leads on which ones are worth pouring resources into targeting.
Google subsidiary DeepMind made the field’s biggest splash in 2020 with the open-sourced release of AlphaFold, its AI system for predicting protein structures. Nobel laureate Venki Ramakrishnan hailed Deepmind’s work as a “stunning advance” that “would fundamentally change biological research” in a company blog post published in 2020.” Even being able to predict the structure of the 200 million proteins leaves a huge challenge for scientists to determine how drugs would interact with these molecules.That research was later published in Nature and garnered Deepmind CEO Demis Hassabis and lead researcher John Jumper a $3 million Breakthrough Prize.
Last November, the Rives-led group touted its model in a paper published by journal Science as being able to make predictions 60 times faster than AlphaFold, though the predictions are less accurate on average. To date, AI has largely provided mere incremental improvements in drug development efficiency. The eureka moment for text-based generative AI — where technical capabilities improved so suddenly and sharply that it triggered a commercial boom — has not yet happened in biology, and some traditional pharmaceutical firms aren’t convinced it ever will happen.
In April, with the ChatGPT-fueled scramble to commercialize generative AI in full swing, Rives’ team departed Meta. While the tech giant set up its AI arm to pursue a wide range of research, its focus has narrowed as certain projects showed commercial viability; similarly, OpenAI disbanded its robotics team in 2021. The Financial Times, which first reported the protein team’s demise, described it as part of wider layoffs and a strategy shift at the mothership to focus on commercial efforts, like an array of AI chatbots. AI for biology has a little prospect of providing lucrative business returns in the immediate term. Commercial players like Schrodinger, which currently trades publicly at a market cap below $3 billion, are selling products based on older school methods of molecular modeling.
EvolutionaryScale is the latest company to raise capital for transformer-based AI research at a massive valuations. Among the unicorn model development companies are Inflection AI, which raised $1.3 billion in June; Cohere, which announced $270 million in May; and Adept, which closed a $350 million investment in March. This week, Hugging Face, a buzzy AI infrastructure provider, announced a $235 million injection at $4.5 billion valuation (Forbes was first to report last month that the company was raising funds). Many of the deals represent bets that negligible revenue will eventually pick up. Stability AI, which raised $100 million at a $1 billion valuation last year, is struggling to generate revenue, among other challenges, as Forbes reported in a June investigation.
Further technical advances in protein folding AI will also require substantial investment. DeepMind setup a new drug discovery arm Isomorphic Labs in December 2022, while rivals Insitro and NASDAQ-listed Recursion have raised over $1 billion from private and public investors (in the pitch document, EvolutionaryScale described these companies as “potential partners” that it could help enable through its models). Even with that investment, the average time to take a drug from discovery through FDA approval is about 7-10 years.
Rives’ team appears to realize its moonshot status. EvolutionaryScale projects that it will spend $38 million in its first year, with $16 million going to computing power, per the pitch document. Costs multiply from there, up to $161 million in year two and $278 million in year three (with $100 million and $200 million spent on compute, respectively). But throughout the document, the company repeatedly emphasizes that it could take ten years for biology AI models to help design products and therapies.
That is, if their hypothesis is accurate in the first place. The intensive compute budget (and the company name) allude to EvolutionaryScale’s big bet: scaling the AI model — feeding it more data and increasing its size — will yield a “capability breakthrough in AI for biology,” the pitch memo states, comparing the current state to that of natural language processing in 2018. “We don’t currently see any other credible efforts focused on the scaling hypothesis in biology,” the document says.
EvolutionaryScale is aiming to build a new model each year, according to the memo. By year three, its ambition is to expand beyond just predicting protein structures to integrating other biological data from DNA sequences, gene expression and epigenetic states. Its long-term vision entails selling a general purpose AI model for biology not specific to any one use case. The model, it theorizes, could be used for medicine — like developing “programmable cells that seek out and destroy cancer or other disease” — but also other biotech applications such as designing “molecular machines” to clean up toxic waste or capture carbon (but this is another challenging market: such an idea mirrors the thesis of Ginkgo Bioworks, which is currently trading publicly 84% below its IPO stock price).
To get there, EvolutionaryScale must prove its advantage over AlphaFold, still the 800-pound gorilla in the sector. Other companies are emerging to tackle similar goals, including Inceptive, which is aiming to apply large language models to design RNA-based drug therapies. The startup was cofounded by Jakob Uszkoreit, a coauthor on the landmark research paper that invented the transformer, the technical breakthrough behind today’s generative AI funding frenzy. One biotech investor who spoke to Forbes anonymously speculated that future versions of more generalized large language models, like the OpenAI’s GPT series, could become skilled enough to be applied to biology.
Such competitive battles likely won’t be a full-time concern for Rives, who is described in the document as “interim CEO.” According to the pitch document, he has an offer to join the faculty at MIT and Harvard’s Broad Institute next year and build out a “biological design lab.”
Alex Konrad contributed reporting.
Denial of responsibility! TechCodex is an automatic aggregator of the all world’s media. In each content, the hyperlink to the primary source is specified. All trademarks belong to their rightful owners, and all materials to their authors. For any complaint, please reach us at – [email protected]. We will take necessary action within 24 hours.
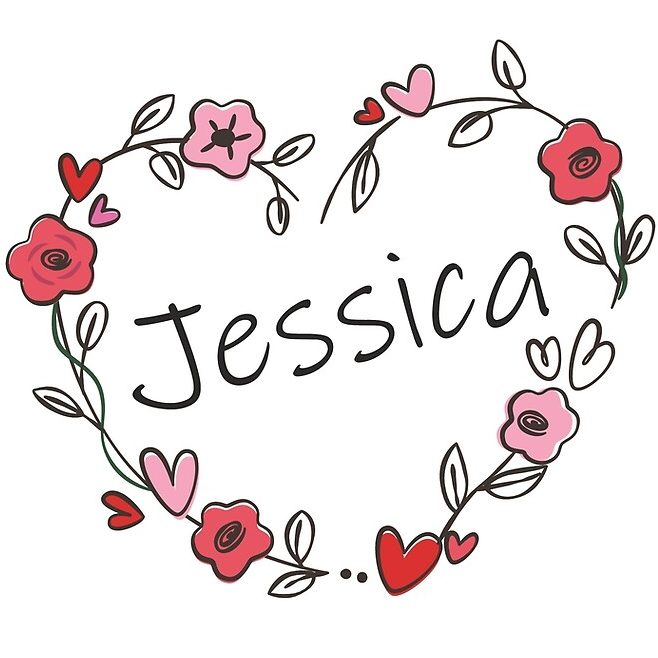
Jessica Irvine is a tech enthusiast specializing in gadgets. From smart home devices to cutting-edge electronics, Jessica explores the world of consumer tech, offering readers comprehensive reviews, hands-on experiences, and expert insights into the coolest and most innovative gadgets on the market.