Patients
SPN patients were collected retrospectively at the Department of Thoracic Surgery of Jiangsu Cancer Hospital from September 2021 to March 2023. The inclusion criteria of this study subjects were as follows: â benign and malignant SPNs confirmed by histopathology after surgery or biopsy and â¡ preoperative DLCT chest enhancement examination. The exclusion criteria were as follows: â pure ground glass or subsolid nodules (containing ground glass components) (nâ=â11); â¡ a history of malignant tumors (nâ=â5); ⢠poor CT image quality due to the lack of continuous thin-layer images or artifacts (nâ=â7); ⣠the number of pulmonary nodules is more than one or primary nodules with several scattered lesions (nâ=â13); ⤠a history of clinical antitumor treatment (nâ=â16); ⥠lesions smaller than 10 mm (nâ=â8) and ⦠nodules with poorly defined boundaries leading to poor segmentation (nâ=â4). After screening patients according to the above criteria, 250 patients with SPN were eventually enrolled in this study for follow-up analysis.
This retrospective study was approved by the Ethics Committee of Jiangsu Cancer Hospital (ethics number: 2023-048), and the experiment was conducted in strict accordance with the ethical standards set out in the 1964 Declaration of Helsinki and its subsequent amendments. The Ethics Committee of Jiangsu Cancer Hospital waived the written informed consent of the patients.
DLCT image acquisition
All patients with SPN were examined by DLCT (IQon, Philips Healthcare, Best, The Netherlands), and breathing training was performed on each patient before scanning. With the patients lying supine on the scanning table, scan from the thoracic inlet to the bottom of the chest to cover all lung tissue. The contrast agent (ioversol, iodine 350 mg/mL, Hengrui Medicine, Lianyungang, China) was injected into the right elbow vein, and then the tube was flushed with 20 ml of normal saline. The injection rate was in the range of 2.5â3.0 ml/s, and the image acquisition during the enhancement period was delayed by 50 s after the injection was completed. The scanning slice thickness of all images was 5 mm and the reconstructed slice thickness was 1 mm. Other parameters were as follows: matrix, 512âÃâ512; collimator width, 64âÃâ0.625 mm; tube current automatic modulation; rotation time, 0.50 s; tube voltage, 120 kVp; scanning field of view, 372 mm; pitch, 0.900.
DLCT image quantitative features
All images were processed and analyzed on the Philips workstation (IntelliSpace Portal, Philips Healthcare). A radiologist used the workstation’s built-in software (Spectral CT Viewer, Philips Healthcare) to delineate the circular regions of interest (ROIs) in the mediastinal window images and perform quantitative analysis, while another senior radiologist supervised from the sidelines. Before the analysis, neither doctor was informed of the clinical data and the pathological diagnosis results of benign and malignant SPN. Two examples (one SPN benign and the other SPN malignant) are shown in Fig. 3. ROIs should cover the areas of lesions with uniform density on the enhanced images to the greatest possible extent, avoiding calcification, blood vessels and necrotic areas. To ensure the stability of the results, the ROIs were drawn on the largest layer of the lesion cross-section and the layers above and below it, and the average of the three measurements was taken as the final analysis data. At the same time, a circular ROI was placed on the aorta with the largest cross-section of the lesion for the standardization of quantitative parameters. Then, other quantitative parameters of the same ROI were obtained on the VNC images, Zeff images, IC images, ED images, CaS images, and 40 keV and 70 keV monoenergetic images.
A 41-year-old male patient with inflammatory pseudotumor in the upper lobe of the left lung (aâd) and a 64-year-old male patient with lung adenocarcinoma (eâh), both pathologically confirmed. a and e are enhanced phase CT images, and the CT values of the lesions are 73.4 HU and 86.9 HU respectively; (b, f) are Zeff images with Zeff values of 8.11 and 8.45 respectively; (c, g) are iodine images, and the IC values are 1.42 mg/ml and 2.17 mg/ml respectively; (d, h) are postoperative pathological pictures.
The quantitative parameters obtained from the ROIs delineated on the lesions and aorta were as follows: CTSPN_VNC, CTSPN_40 keV, CTSPN_70 keV, CT values of aorta on VNC images (CTaorta_VNC) and CT values of 70 keV after aortic enhancement (CTaorta). Derived parameters were calculated based on the above values, including SARVNC, SAR40 keV, SAR70 keV, Î40 keV, Î70 keV, ÎSA_40 keV, ÎSA_70 keV, CER40 keV, CER70 keV, NEF40 keV, NEF70 keV and λHU. The calculation formulas of the above derived parameters were as follows30,31,32,33,34:
$${\text{SAR}}_{{{\text{VNC}}}} = {\text{ CT}}_{{{\text{SPN}}\_{\text{VNC}}}} /{\text{CT}}_{{{\text{aorta}}\_{\text{VNC}}}}$$
$${\text{SAR}}_{{{4}0\;{\text{keV}}}} = {\text{ CT}}_{{{\text{SPN}}\_{4}0\;{\text{keV}}}} /{\text{CT}}_{{{\text{aorta}}}}$$
$${\text{SAR}}_{{{7}0\;{\text{keV}}}} = {\text{CT}}_{{{\text{SPN}}\_{7}0\;{\text{keV}}}} /{\text{CT}}_{{{\text{aorta}}}}$$
$$\Delta_{{{4}0\;{\text{keV}}}} = {\text{ CT}}_{{{\text{SPN}}\_{4}0\;{\text{keV}}}} {-}{\text{CT}}_{{{\text{SPN}}\_{\text{VNC}}}}$$
$$\Delta_{{{7}0\;{\text{keV}}}} = {\text{CT}}_{{{\text{SPN}}\_{7}0\;{\text{keV}}}} {-}{\text{CT}}_{{{\text{SPN}}\_{\text{VNC}}}}$$
$$\Delta_{{{\text{SA}}\_{4}0\;{\text{keV}}}} = {\text{CT}}_{{{\text{SPN}}\_{4}0\;{\text{keV}}}} {-}{\text{CT}}_{{{\text{aorta}}}}$$
$$\Delta_{{{\text{SA}}\_{7}0\;{\text{keV}}}} = {\text{CT}}_{{{\text{SPN}}\_{7}0\;{\text{keV}}}} {-}{\text{CT}}_{{{\text{aorta}}}}$$
$${\text{CER}}_{{{4}0\;{\text{keV}}}} = \Delta_{{{4}0\;{\text{keV}}}} /{\text{CT}}_{{{\text{SPN}}\_{\text{VNC}}}}$$
$${\text{CER}}_{{{7}0\;{\text{keV}}}} = \Delta_{{{7}0\;{\text{keV}}}} /{\text{CT}}_{{{\text{SPN}}\_{\text{VNC}}}}$$
$${\text{NEF}}_{{{4}0\;{\text{keV}}}} = \Delta_{{{4}0\;{\text{keV}}}} /\left( {{\text{CT}}_{{{\text{aorta}}}} {-}{\text{CT}}_{{{\text{aorta}}\_{\text{VNC}}}} } \right)$$
$${\text{NEF}}_{{{7}0\;{\text{keV}}}} = \Delta_{{{7}0\;{\text{keV}}}} /\left( {{\text{CT}}_{{{\text{aorta}}}} {-}{\text{CT}}_{{{\text{aorta}}\_{\text{VNC}}}} } \right)$$
$$\uplambda _{{{\text{HU}}}} = \left( {{\text{CT}}_{{{\text{SPN}}\_{4}0\;{\text{keV}}}} {-}{\text{CT}}_{{{\text{SPN}}\_{7}0\;{\text{keV}}}} } \right)/\left( {{7}0{-}{4}0} \right)$$
Considering the differences in cardiac function and hemodynamics between patients, the IC automatically measured on the iodine image was normalized to the aorta, the NIC was calculated, and the NCaS, NED and NZeff were calculated in the same way. The calculation formulas were as follows:
$${\text{NIC}} = {\text{IC}}_{{{\text{SPN}}}} /{\text{IC}}_{{{\text{aorta}}}}$$
$${\text{NCaS}} = {\text{CaS}}_{{{\text{SPN}}}} /{\text{CaS}}_{{{\text{aorta}}}}$$
$${\text{NED}} = {\text{ED}}_{{{\text{SPN}}}} /{\text{ED}}_{{{\text{aorta}}}}$$
$${\text{NZeff}} = {\text{Zeff}}_{{{\text{SPN}}}} /{\text{Zeff}}_{{{\text{aorta}}}}$$
Machine learning model
All SPN patients were randomly divided into the training and test sets by 7ê3 and the patients in the training set were balanced at a ratio of 1:1 by using the synthetic minority oversampling technique, so that the number of benign SPN patients and malignant SPN patients was consistent35,36. The LASSO algorithm was used to screen features from patientsâ clinical data and DLCT parameters, and 6 classical ML models were constructed in the training set based on the selected features, namely AdaBoost, GNB, LR, RF, SVM and XGBoost37. The ROC curves of the 6 models were plotted to obtain the AUC of the respective models, and the accuracy, sensitivity and specificity were calculated simultaneously to serve as the evaluation indicators for the 6 models. The models were evaluated by tenfold cross-validation, and the performance of the models was further validated using test set data. The above modeling process was based on R software (version 4.2.3) and Python programming language (version 3.11.4).
Statistical analysis
The ShapiroâWilk test was employed to assess the normal distribution of the data. The independent sample t-test was utilized for comparing the features of continuous data with a normal distribution, while the MannâWhitney U test was used for comparing the features of continuous data with a non-normal distribution. The chi-square test or Fisherâs exact test was applied to compare the characteristics of count data. The statistical analysis of patients’ clinical data and DLCT parameters was conducted using SPSS Statistics 26.0 (IBM Corp., Chicago, Illinois, United States of America) software, with statistical significance indicated by Pâ<â0.05.
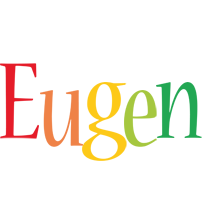
Eugen Boglaru is an AI aficionado covering the fascinating and rapidly advancing field of Artificial Intelligence. From machine learning breakthroughs to ethical considerations, Eugen provides readers with a deep dive into the world of AI, demystifying complex concepts and exploring the transformative impact of intelligent technologies.