Ki-67 serves as a crucial indicator for predicting cancer recurrence and survival among early-stage high-risk breast cancer patients1,2. It informs decisions regarding adjuvant chemotherapy12 and radiation therapy opt-out for Luminal A breast cancer patients13. These clinical decisions often rely on PI scores between 5 and 30%; however, this range exhibits significant scoring variability among experts, making standardization and clinical application challenging8,9,12. This inconsistency, combined with long assessment times using the current Ki-67 scoring system, has limited the broader clinical application of Ki-67 and resultantly, has not yet been integrated into all clinical workflows16. AI technologies are being proposed to improve Ki-67 scoring accuracy, inter-rater agreement, and TAT. This study explores the influence of AI in these three areas by recruiting 90 pathologists to examine ten breast cancer TMAs with PIs in the range of 7 to 28%.
Two previous studies aimed to quantify PI accuracy with and without AI19,23. One study demonstrated that AI-enhanced microscopes improved invasive breast cancer assessment accuracy23. They had 30 pathologists use an AI microscope to evaluate 100 invasive ductal carcinoma IHC-stained whole slide images (WSIs), which provided tumor delineations, and cell annotations. AI use resulted in a mean PI error reduction from 9.60 to 4.53. A similar study was conducted19, where eight pathologists assessed 200 regions of interest using an AI tool. Pathologists identified hotspots on WSIs, after which the AI tool provided cell annotations for the clinician’s review. The study found that this method significantly improved the accuracy of Ki-67 PI compared to traditional scoring (14.9 error without AI vs. 6.9 error with AI).
Similarly, this study found that using AI assistance for PI scoring significantly (p < 0.001) improved pathologists’ accuracy, reducing both the PI error and its standard deviation across various demographics, including years of experience and specialties. This indicates that AI assistance leads to higher PI accuracy across all levels of pathologists’ training, enabling professionals at every career stage to deliver more precise PI scores in the range critical for clinical decision-making. This improvement may help bridge experience gaps and is critical for PI scoring standardization. An underestimation trend, previously reported by33, was also noted in this study, as shown by the PI correlation and Bland–Altman analysis (Fig. 3). However, scoring with the support of AI improved PI accuracy for all cases and corrected this underestimation bias. This is exemplified by the scoring near the 20% cutoff, which simulates a clinical decision threshold. In conventional assessments, many pathologists select the incorrect range (≥ 20% or < 20%), particularly for TMAs 7, 8, and 9, with ground truths of 19.8, 23.7, and 28.2, respectively. For instance, TMA 8 had 76.7% of respondents incorrectly estimated the score as < 20%. Errors like these would result in incorrect therapy decisions and poor patient outcomes. Fortunately, with AI assistance, the percentage of pathologists agreeing with the ground truth greatly improved, providing a strong incentive for the clinical use of AI tools in Ki-67 scoring. All cases showed a statistically significant PI error decrease with AI assistance, except for Case 1, with a ground truth PI score of 7.3% (p = 0.133). This exception could be attributed to fewer Ki-67 positive cells requiring counting, which likely simplified the scoring process.
In addition to accuracy, PI scoring agreement is critical to ensure that patients with similar disease phenotypes are delivered the proper therapeutic regimes. However, significant variability in Ki-67 scoring is widely recognized, even in established laboratories. A study led by34, found reproducibility among eight labs was only moderately reliable with contributing factors such as subjective judgements related to PI scoring and tumor region selection. Standardizing scoring methods becomes imperative, as transferring Ki-67 PIs and cutoffs between laboratories would compromise analytical validity. In another study by35, the variability in breast cancer biomarker assessments, including Ki-67, among pathology departments in Sweden was investigated. While positivity rates for HR and HER2 had low variability, there was substantial variation in Ki-67 scoring, where 66% of labs showed significant intra-laboratory variability. This variability could potentially affect the distribution of endocrine and HER2-targeted treatments, emphasizing the need for improved scoring methods to ensure consistent and dependable clinical decision-making. The study by23, aimed to improve Ki67 scoring concordance with their AI-empowered microscope. They found a higher ICC of 0.930 (95% CI: 0.91–0.95) with AI, compared to 0.827 (95% CI: 0.79–0.87) without AI. Similarly22, aimed to quantify the inter-rater agreement for WSIs with AI assistance across various clinical settings. The AI tool evaluated 72 Ki-67 breast cancer slides by annotating Ki-67 cells and providing PI scores. Ten pathologists from eight institutes reviewed the tool and input their potentially differing PI scores. When the scores were categorized using a PI cutoff of 20%, there was an 87.6% agreement between traditional and AI-assisted methods. Results also revealed a Krippendorff’s α of 0.69 in conventional eyeballing quantification and 0.72 with AI assistance indicative of increased inter-rater agreement, however, these findings were not significant.
In this study, we evaluated the scoring agreement with and without AI across 90 pathologists, representing one of the largest cohorts analyzed for this task. It was found that over the critical PI range of 7 to 28%, AI improved the inter-rater agreement, with superior ICC, Krippendorff’s α and Fleiss’ Kappa values compared to conventional assessments and higher correlation of PI estimates with the ground truth PI score. Additionally, there was a decrease in offset and variability, as shown in Fig. 3. These agreement metrics align with findings from earlier studies22,23 and signify that AI tools can standardize Ki-67 scoring, enhance reproducibility and reduce the subjective differences seen with conventional assessments. Therefore, using an AI tool for Ki-67 scoring could lead to more robust assessments and consistent therapeutic decisions.
AI applications have predominantly focused on automating the laborious tasks for pathologists, thereby freeing up time for high-level, critical decision-making, especially those related to more complex disease presentations16,17,18,19,20,36. Some research into AI support tools in this field has demonstrated a notable decrease in TAT for pathologists. For instance, a study led by37, which involved 20 pathologists analyzing 240 prostate biopsies, reported that an AI-based assistive tool significantly reduced TAT, with 13.5% less time spent on assisted reviews than on unassisted ones. Similarly, the study by38, demonstrated a statistical improvement (p < 0.05) in TATs when 24 raters counted breast mitotic figures in 140 high-power fields, with and without AI support, ultimately achieving a time saving of 27.8%. However, the study by23, reported a longer TAT using an AI-empowered microscope in their study, which involved 100 invasive ductal carcinoma WSIs and 30 pathologists (11.6 s without AI vs. 23.8 s with AI).
Our study found that AI support resulted in faster TATs (18.3 s without AI vs. 16.8 s with AI, p < 0.001), equating to a median time saving of 11.9%. Currently, our team only performs Ki-67 testing upon oncologists’ requests, as routine Ki-67 assessment is not yet standard practice. This is partly due to the difficulties in standardizing Ki-67, compounded by pathologists’ increasing workloads and concerns over burnout39,40. Pathologists’ caseloads have grown in the past decade, from 109 to 116 annually in Canada and 92 to 132 in the U.S.41. With the Canadian Cancer Society expecting 29,400 breast cancer cases in 202342, routine Ki-67 assessments would significantly increase workloads. Therefore, the implementation of AI tools in this context could alleviate workload pressures by offering substantial time savings and supporting the clinical application of this important biomarker.
The gold standard for assessing Ki-67 PI is manual counting8,9; however, due to the labor-intensive nature of this method, many pathologists often resort to rough visual estimations43,44. As indicated in Table 3 and Fig. 4, the shorter TATs suggest that respondents may have relied on visual estimations for Ki-67 scoring. Despite this, the TATs significantly improved (p < 0.001) when using AI. This improvement was evident among experienced pathologists; however, some encountered longer TATs after integrating AI, possibly due to unfamiliarity with the AI tool or digital pathology viewing software. Although participants received a brief orientation and two initial examples, the novelty of the tool might have posed a learning curve. Addressing this challenge involves integrating the tool into regular practice and providing comprehensive training before its use.
The perspectives of pathologists highlight a growing enthusiasm towards AI integration for Ki-67 evaluations for breast cancer. A significant 84% of participants agreed the AI’s recommendations were suitable for the task at hand. They recognized AI’s ability to improve pathologists’ accuracy (76%), enhance inter-rater consistency (82%), and reduce the TAT for Ki-67 evaluations (83%). Additionally, 49% expressed their intent to incorporate AI into their workflow, and 47% anticipated the routine implementation of AI within the next decade. An important observation is that many respondents who were hesitant about personally or routinely implementing AI in clinical practice were retired pathologists. In total, 83% of retired pathologists reported they would not currently implement AI personally or routinely, which is a stark contrast to only 15% of practicing pathologists who expressed the same reluctance. This positive outlook in the pathologist community supports the insights of this study and signals an increasing momentum for the widespread adoption of AI into digital pathology.
The strength of this research is highlighted by the extensive and diverse participation of 90 pathologists, which contributes to the study’s generalizability in real-world clinical contexts. Adding to the study’s credibility is the focus on Ki-67 values around the critical 20% threshold, which is used for adjuvant therapy decisions. Moreover, the AI nuclei overlay addresses the transparency concerns often associated with AI-generated scores, thus improving clarity and comprehensibility for users. The ongoing discussion around ‘explainable AI’ highlights the importance of transparency in AI tools’ outputs, a crucial factor for their acceptance and adoption45. The outcomes of the study emphasize the positive outlook and readiness of pathologists to embrace AI in their workflow and serve to reinforce the growing need for the integration of AI into regular medical practice.
The study has its limitations, one of which includes the potential unintentional inclusion of non-pathologists. The survey required respondents to confirm their status as pathologists through agreement before beginning; however, due to confidentiality limitations, no further verification was possible. In some instances, pathologists’ scores deviated from the ground truth by more than 20%, with PI errors reaching up to 50%. Such large errors would render any PI score diagnostically irrelevant, as the variance exceeds the clinical threshold of 20%. These errors might be attributed to input errors or a lack of experience in Ki-67 assessments. Consequently, we used this threshold to filter out potentially erroneous responses. In total, 26 participants who logged responses exceeding the 20% error threshold were subsequently excluded from the study. For completeness, Supplementary Table 2 discloses the PI scores and PI errors of all respondents, including outliers, where the data trends appear similar. The demographics of the study’s participants reveal there was limited participation from currently practicing pathologists, representing 14.4% of respondents. This may be attributed to the time constraints faced by practicing pathologists. In future research, efforts will be made to include more practicing pathologists and to evaluate intra-observer variability. Additionally, while the survey provided specific guidelines for calculating the PI and applying Ki-67 positivity criteria, the accuracy and thoroughness of each pathologist’s evaluations could not be verified. Lastly, the study deviated from standard practice by using TMAs instead of WSIs for Ki-67 clinical assessments. The rationale behind this choice was the expectation of more precise scoring with TMAs, as this eliminates the need to select high-power fields (a subjective process) and involves a lower number of cells to evaluate, leading to better consistency in visual estimations. Future research should focus on evaluating the accuracy achieved with AI assistance in identifying regions of interest and analyzing WSIs. This should also incorporate a broader range of cases and a wider PI range. Prospective studies involving solely practicing breast pathologists could also yield valuable insights into the real-world application of the AI tool and its impact on clinical decision-making.
In conclusion, this study provides early insights into the potential of an AI tool in improving the accuracy, inter-rater agreement, and workflow efficiency of Ki-67 assessment in breast cancer. As AI tools become more widely adopted, ongoing evaluation and refinement will be essential to fully realize its potential and optimize patient care. Such tools are critical for robustly analyzing large datasets and effectively determining PI thresholds for treatment decisions.
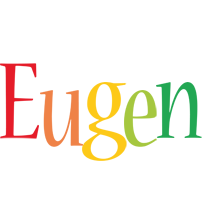
Eugen Boglaru is an AI aficionado covering the fascinating and rapidly advancing field of Artificial Intelligence. From machine learning breakthroughs to ethical considerations, Eugen provides readers with a deep dive into the world of AI, demystifying complex concepts and exploring the transformative impact of intelligent technologies.