Scientists at UC San Francisco have developed a machine learning model capable of predicting the onset of Alzheimer’s disease up to seven years before clinical symptoms manifest. By analyzing electronic health records, the team identified high cholesterol and osteoporosis, particularly in women, as significant predictors of Alzheimer’s.
This research, published on February 21, 2024, in Nature Aging, underscores the potential of artificial intelligence (AI) to revolutionize early diagnosis and understanding of complex diseases like Alzheimer’s disease.
“This is a first step towards using AI on routine clinical data, not only to identify risk as early as possible, but also to understand the biology behind it,” said the study’s lead author, Alice Tang, an MD/PhD student in the Sirota Lab at UCSF. “The power of this AI approach comes from identifying risk based on combinations of diseases.”
Alzheimer’s disease stands as the most prevalent form of dementia, particularly affecting those over the age of 65. It is characterized by progressive memory loss, cognitive decline, and a variety of neurological changes, including the accumulation of amyloid-beta plaques and tau tangles in the brain. These pathological changes disrupt the normal functioning of neural cells, leading to the symptoms and eventual severe disability associated with the disease.
Despite ongoing research, there remains no cure for Alzheimer’s, with current treatments largely focused on managing symptoms rather than halting or reversing the disease’s progression.
Early detection of Alzheimer’s disease offers a pivotal advantage: the potential for earlier intervention, which could significantly alter the disease’s trajectory or mitigate its impacts. Traditional methods for diagnosing Alzheimer’s disease — ranging from cognitive assessments to biomarker analysis — are often applied only after symptoms have manifested, which may be too late for optimal therapeutic interventions.
To develop their predictive models, the research team leveraged the UCSF Medical Center’s extensive electronic health databases. From this pool, researchers identified 749 individuals with Alzheimer’s disease, based on expert-level clinical diagnoses, and 250,545 controls without a dementia diagnosis.
The methodology hinged on the use of Random Forest (RF) models, a type of machine learning algorithm suitable for handling the complex, non-linear relationships often present in medical data. The models were trained using a comprehensive range of clinical data points extracted from the electronic health records, including demographics, disease conditions, drug exposures, and abnormal laboratory measures.
The findings revealed that the machine learning models could accurately predict Alzheimer’s disease onset with significant reliability (72%), up to seven years in advance. The inclusion of demographic and visit-related features alongside clinical data further enhanced the models’ predictive accuracy.
Several factors, including hypertension, high cholesterol and vitamin D deficiency, emerged as top predictors of Alzheimer’s disease in both men and women. Erectile dysfunction and an enlarged prostate were also predictive for men. For women, the study highlighted osteoporosis as an additional significant predictor, suggesting a gender-specific pathway or vulnerability to the disease.
But not every woman suffering from osteoporosis is destined to develop Alzheimer’s disease. “It is the combination of diseases that allows our model to predict Alzheimer’s onset,” said Tang, “Our finding that osteoporosis is one predictive factor for females highlights the biological interplay between bone health and dementia risk.”
To delve deeper into the biological mechanisms that underpin the predictive capabilities of their model, the researchers utilized public molecular databases along with a powerful tool developed at UCSF known as SPOKE (Scalable Precision Medicine Oriented Knowledge Engine).
Developed in the laboratory of Sergio Baranzini, a professor of neurology and a member of the UCSF Weill Institute for Neurosciences, SPOKE is designed as a “database of databases.” This innovative tool enables researchers to sift through vast amounts of data to uncover patterns and identify potential molecular targets for therapeutic intervention.
SPOKE confirmed the link between Alzheimer’s disease and high cholesterol via the APOE4 variant of the apolipoprotein E gene. This association is widely recognized in the scientific community. However, the integration of SPOKE with genetic databases yielded a novel insight, uncovering a connection between osteoporosis and Alzheimer’s specifically in women.
This link was identified through a variant in the MS4A6A gene, which is less well-known in the context of Alzheimer’s research. The discovery of this association exemplifies the strength of combining advanced computational tools like SPOKE with extensive genetic data, paving the way for targeted research into the molecular pathways involved in Alzheimer’s and potentially guiding the development of new therapeutic strategies.
The findings represent a significant advancement in the fight against Alzheimer’s disease. But, despite the promising results, the study has a few limitations, including the challenges of interpreting electronic health record data, the potential for cohort selection biases, and the need for continuous model retraining to adapt to changing clinical practices. The study’s predictive models need to be validated in broader and more diverse populations to ensure their accuracy and generalizability.
The researchers are optimistic that their methods could be applied to other diseases that are challenging to diagnose, such as lupus and endometriosis.
“This is a great example of how we can leverage patient data with machine learning to predict which patients are more likely to develop Alzheimer’s, and also to understand the reasons why that is so,” said the study’s senior author, Marina Sirota, an associate professor at the Bakar Computational Health Sciences Institute at UCSF.
The study, “Leveraging electronic health records and knowledge networks for Alzheimer’s disease prediction and sex-specific biological insights,” was authored by Alice S. Tang, Katherine P. Rankin, Gabriel Cerono, Silvia Miramontes, Hunter Mills, Jacquelyn Roger, Billy Zeng, Charlotte Nelson, Karthik Soman, Sarah Woldemariam, Yaqiao Li, Albert Lee, Riley Bove, Maria Glymour, Nima Aghaeepour, Tomiko T. Oskotsky, Zachary Miller, Isabel E. Allen, Stephan J. Sanders, Sergio Baranzini, and Marina Sirota.
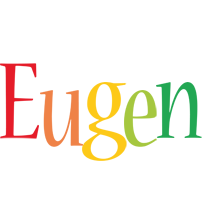
Eugen Boglaru is an AI aficionado covering the fascinating and rapidly advancing field of Artificial Intelligence. From machine learning breakthroughs to ethical considerations, Eugen provides readers with a deep dive into the world of AI, demystifying complex concepts and exploring the transformative impact of intelligent technologies.