• Physics 17, 13
Researchers have used quantum computers to solve difficult physics problems. But claims of a quantum “advantage” must wait as ever-improving algorithms boost the performance of classical computers.
TechSolution/stock.adobe.com
Quantum computers have plenty of potential as tools for carrying out complex calculations. But exactly when their abilities will surpass those of their classical counterparts is an ongoing debate. Recently, a 127-qubit quantum computer was used to calculate the dynamics of an array of tiny magnets, or spins—a problem that would take an unfathomably long time to solve exactly with a classical computer [1]. The team behind the feat showed that their quantum computation was more accurate than nonexact classical simulations using state-of-the-art approximation methods. But these methods represented only a small handful of those available to classical-computing researchers. Now Joseph Tindall and his colleagues at the Flatiron Institute in New York show that a classical computer using an algorithm based on a so-called tensor network can produce highly accurate solutions to the spin problem with relative ease [2]. The results show that classical computers still have many tricks up their sleeves, making it hard to predict when quantum computers will gain the upper hand.
Quantum computers have made great leaps in performance, leading to natural comparisons with classical computers. In 2019, Google’s 53-qubit Sycamore quantum computer took 200 seconds to perform a specific computation that was predicted to take 10,000 years with a classical computer, leading researchers to claim that their system had a quantum advantage [3]. Other groups immediately countered the claim, noting various ways that they could accelerate classical methods, reducing the presumed advantage of Google’s quantum technique (see Viewpoint: Imperfections Lower the Simulation Cost of Quantum Computers). “Quantum computing is not the only thing that’s improving,” Tindall says. “Classical methods are also improving and have been improving for decades.”
Researchers are now shying away from direct quantum–classical rivalry, instead focusing on where quantum computers can prove useful. “There’s this unfortunate emphasis on immediately trying to show an advantage,” says IBM quantum-computing expert Abhinav Kandala. Rather than advantage, he says that the first thing to show is “utility,” which is defined as a quantum computer giving an accurate solution to a problem that is beyond exact classical computation. Achieving utility has been challenging, given that quantum computers are currently noisy and prone to errors. Kandala and his colleagues demonstrated quantum utility in the summer of last year using IBM’s 127-qubit Eagle quantum computer to solve a common type of physics problem based on the so-called Ising model [1].
The Ising model concerns a collection of spins that interact with each other, affecting their mutual alignment. Condensed-matter physicists often use the Ising model to study magnetic phenomena in materials, but it becomes increasingly difficult to solve Ising-based problems as the number of spins increases. Focusing on a specific spin system, Kandala and his colleagues used their quantum computer to determine quantities such as the system’s overall spin alignment, or magnetization. They then developed a noise-mitigation strategy to extrapolate the predictions to zero-error solutions, which they compared to exact solutions that were available for certain values of the input variables. Via this comparison, the researchers showed that their quantum computations were more accurate than predictions obtained from classical simulations performed by team members from the University of California, Berkeley, on a supercomputer.
The news of this quantum-utility demonstration spread fast. “It was a big deal,” Tindall says. But after looking over the result, he and his colleagues wondered if the IBM team had perhaps sold short the capabilities of classical methods in their accuracy comparison. Tindall’s team now reports an improved classical simulation method that better measures up against the quantum one used by Kandala’s team.
The new classical method uses tensor networks—series of data arrays connected through links. Physicists have long used tensor networks to study many-body quantum systems, such as the electrons in a superconductor or the atoms in a molecule. The networks allow them to compress the enormous amounts of information contained in a full description of the wave function of such a system. “A tensor network is essentially like a zip file for the wave function,” Tindall says.
Tindall and his colleagues designed a “zip file” that simulates the 127 qubits in IBM’s computer. To fully represent the wave function of these qubits would require 2127≈ 1038 numbers, which in bytes would be trillions and trillions of times more data than are stored on all the computers in the world. The researchers reduced the amount of data needed to less than about a billion numbers by assuming that some of the wave function’s information—specifically some of the information about the quantum entanglement between qubits—could be neglected.
Applying their network to the Ising-model problem, Tindall and his colleagues solved the problem on a classical computer with greater accuracy than achieved using the quantum one. Tindall notes that Kandala and his colleagues also performed tensor-network computations as part of their classical comparison. But he says that the network they chose wasn’t explicitly designed to have the same geometry as their computer. Making a different choice meant that Tindall and his colleagues did not require a supercomputer to run their tests. “You could run some of the simulations on a mobile phone,” he says.
Tindall and his colleagues “have successfully applied a novel classical algorithm to a timely problem that is of relevance to a large part of the physics community,” says Michael Lubasch, a scientist at the quantum-computing company Quantinuum. But Lubasch stresses that the classical algorithm the team used is tailored to work for a particular Ising-model problem. Researchers cannot use this algorithm to simulate every computation that a quantum computer can do, he says.
Kandala is unsurprised that a classical method met the bar of their quantum computation. “This was exactly the kind of response we were hoping for,” he says. He calls the back and forth between the quantum- and classical-computing communities a “symbiotic relationship,” in which the two sides challenge each other by developing ever-more sophisticated computing methods. “Hopefully we can work together to figure out the challenging problems that will take us from utility to advantage,” he says. Tindall imagines that such a problem will eventually appear, but he has not committed a time frame for that appearance. “It could be a very long time from now,” he says.
–Michael Schirber
Michael Schirber is a Corresponding Editor for Physics Magazine based in Lyon, France.
References
- Y. Kim et al., “Evidence for the utility of quantum computing before fault tolerance,” Nature 618, 500 (2023).
- J. Tindall et al., “Efficient tensor network simulation of IBM’s Eagle kicked Ising experiment,” PRX Quantum 5, 010308 (2024).
- F. Arute et al., “Quantum supremacy using a programmable superconducting processor,” Nature 574, 505 (2019).
Subject Areas
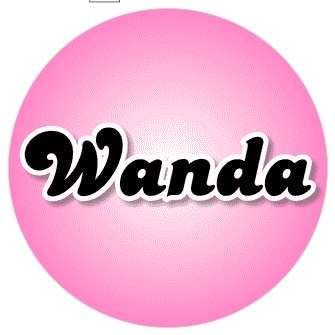
Wanda Parisien is a computing expert who navigates the vast landscape of hardware and software. With a focus on computer technology, software development, and industry trends, Wanda delivers informative content, tutorials, and analyses to keep readers updated on the latest in the world of computing.