A groundbreaking study conducted by Cornell researchers has demonstrated the successful application of data science and artificial intelligence (AI) tools in identifying discriminatory practices employed by prosecutors during the jury selection process. The study specifically focuses on instances where prosecutors intentionally sought to exclude women and Black individuals from serving on juries.
Using natural language processing (NLP) tools, the researchers analyzed transcripts of the jury selection process and discovered numerous quantifiable discrepancies in how prosecutors questioned Black and white members of the jury pool. This technology, once validated, has the potential to provide crucial evidence for appeals cases and can be utilized in real-time during jury selection to ensure greater diversity in juries.
The study, titled “Quantifying Disparate Questioning of Black and White Jurors in Capital Jury Selection,” was published in the Journal of Empirical Legal Studies on July 14. Anna Effenberger is the first author of the study.
Although it has been illegal since the 1986 Supreme Court case Batson vs. Kentucky to exclude jurors based on race or gender, this form of discrimination continues to persist. John Blume, co-author of the study and the Samuel F. Leibowitz Professor of Trial Techniques at Cornell Law School, emphasizes the importance of examining whether prosecutors exhibit different questioning patterns towards Black and white jurors. The utilization of NLP software allows for a more sophisticated analysis of these patterns beyond just the quantity of questions asked.
In instances where prosecutors perceive Black and female jurors as potentially more sympathetic towards defendants, particularly Black defendants, they may employ tactics to elicit disqualifying responses. For example, in capital cases, prosecutors may provide graphic descriptions of the execution process and inquire whether the individual would be comfortable sentencing the defendant to death. If the answer is negative, that person is eliminated from the jury pool.
To determine whether NLP software can identify these discrepancies and other signs of biased questioning, Blume collaborated with Martin Wells, the Charles A. Alexander Professor of Statistical Sciences in the Cornell Ann. S Bowers College of Computing and Information Science, and Effenberger. Together, they analyzed transcripts from 17 capital cases in South Carolina, totaling over 26,000 questions posed by judges, defense attorneys, and prosecutors to potential jurors.
The researchers not only examined the quantity of questions asked to Black, white, male, and female potential jurors, but also the topics covered, complexity of each question, and the parts of speech used. Wells highlights that unlike job interviews where a standardized list of questions is typically utilized, this is not the case during jury selection.
The analysis revealed significant disparities in the length, complexity, and sentiment of the questions posed by prosecutors to Black potential jurors compared to their white counterparts, indicating an intention to shape their responses. Conversely, no racial differences were observed in the questions asked by judges and the defense. The study also presented evidence of prosecutors attempting to disqualify Black individuals based on their views on the death penalty. Specifically, Black potential jurors, especially those ultimately excused from serving, were subjected to more explicit and graphic questions about execution methods when compared to white potential jurors.
Out of the 17 cases analyzed, six were found to have involved illegal removal of potential jurors based on race, as later ruled by a judge. By integrating the NLP analyses for each case, the researchers successfully distinguished between cases that violated the Batson vs. Kentucky ruling and those that did not.
These findings establish the capability of NLP tools to identify biased jury selection and pave the way for future studies involving larger datasets and diverse types of cases. Once the reliability of this method is established, Wells envisions its potential usage in real-time during jury selection to monitor and prevent discrimination.
Whether utilized to ensure diverse juries or provide evidence during appeals, this software can become a powerful tool, particularly for defendants facing the death penalty.
More information:
Anna Effenberger et al, Quantifying disparate questioning of Black and White jurors in capital jury selection, Journal of Empirical Legal Studies (2023). DOI: 10.1111/jels.12357
Citation:
Analysis of court transcripts reveals biased jury selection (2023, July 28)
retrieved 28 July 2023
from https://phys.org/news/2023-07-analysis-court-transcripts-reveals-biased.html
This document is subject to copyright. Apart from any fair dealing for the purpose of private study or research, no
part may be reproduced without the written permission. The content is provided for information purposes only.
Denial of responsibility! TechCodex is an automatic aggregator of the all world’s media. In each content, the hyperlink to the primary source is specified. All trademarks belong to their rightful owners, and all materials to their authors. For any complaint, please reach us at – [email protected]. We will take necessary action within 24 hours.
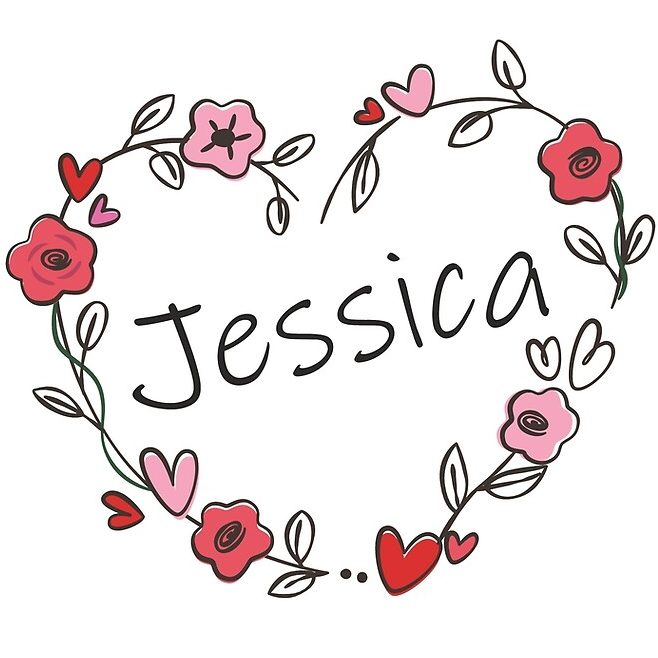
Jessica Irvine is a tech enthusiast specializing in gadgets. From smart home devices to cutting-edge electronics, Jessica explores the world of consumer tech, offering readers comprehensive reviews, hands-on experiences, and expert insights into the coolest and most innovative gadgets on the market.