Chauhan SS, Manfredi MA, Abu Dayyeh BK, Enestvedt BK, Fujii-Lau LL, Komanduri S, et al. Enteroscopy. Gastrointest Endosc. 2015;82(6):975–90.
Kim JS, Kim BW. Training in endoscopy: esophagogastroduodenoscopy. Clin Endosc. 2017;50(4):318–21.
May A. Double-balloon Enteroscopy. Gastrointest Endosc Clin N Am. 2017;27(1):113–22.
Aniwan S, Viriyautsahakul V, Luangsukrerk T, Angsuwatcharakon P, Piyachaturawat P, Kongkam P, et al. Low rate of recurrent bleeding after double-balloon endoscopy-guided therapy in patients with overt obscure gastrointestinal bleeding. Surg Endosc. 2021;35(5):2119–25.
Gomes C, Rubio Mateos JM, Pinho RT, Ponte A, Rodrigues A, Fosado Gayosso M, et al. The rebleeding rate in patients evaluated for obscure gastrointestinal bleeding after negative small bowel findings by device assisted enteroscopy. Rev Esp Enferm Dig. 2020;112(4):262–8.
Hashimoto R, Matsuda T, Nakahori M. False-negative double-balloon enteroscopy in overt small bowel bleeding: long-term follow-up after negative results. Surg Endosc. 2019;33(8):2635–41.
Shinozaki S, Yano T, Sakamoto H, Sunada K, Hayashi Y, Sato H, et al. Long-term outcomes in patients with overt obscure gastrointestinal bleeding after negative double-balloon endoscopy. Dig Dis Sci. 2015;60(12):3691–6.
Guo Y, Liu Y, Oerlemans A, Lao S, Wu S, Lew MS. Deep learning for visual understanding: a review. Neurocomputing. 2016;187:27–48.
Krizhevsky A, Sutskever I, Hinton GE. ImageNet classification with deep convolutional neural networks. Commun ACM. 2017;60(6):84–90.
Kumar P, Manash E. Deep learning: a branch of machine learning. J Phys Conf Ser. 2019;1228:012045.
LeCun Y, Bengio Y, Hinton G. Deep learning. Nature. 2015;521(7553):436–44.
Russell SJ, Norvig P. Artificial intelligence. a modern approach. Third ed. Pearson; 2014.
Bibault J-E, Giraud P, Burgun A. Big data and machine learning in radiation oncology: state of the art and future prospects. Cancer Lett. 2016;382(1):110–7.
Cruz-Roa A, González FA, Gilmore H, Basavanhally A, Feldman M, Shih NNC, et al. Accurate and reproducible invasive breast cancer detection in whole-slide images: a deep learning approach for quantifying tumor extent. Sci Rep. 2017;7:46450.
Ehteshami Bejnordi B, Veta M, Johannes van Diest P, van Ginneken B, Karssemeijer N, Litjens G, et al. Diagnostic assessment of deep learning algorithms for detection of lymph node metastases in women with breast Cancer. Jama. 2017;318(22):2199–210.
Esteva A, Kuprel B, Novoa RA, Ko J, Swetter SM, Blau HM, et al. Dermatologist-level classification of skin cancer with deep neural networks. Nature. 2017;542(7639):115–8.
Gulshan V, Peng L, Coram M, Stumpe MC, Wu D, Narayanaswamy A, et al. Development and validation of a deep learning algorithm for detection of diabetic retinopathy in retinal fundus photographs. Jama. 2016;316(22):2402–10.
Larson DB, Chen MC, Lungren MP, Halabi SS, Stence NV, Langlotz CP. Performance of a deep-learning neural network model in assessing skeletal maturity on pediatric hand radiographs. Radiology. 2018;287(1):313–22.
Ting DSW, Cheung CY, Lim G, Tan GSW, Quang ND, Gan A, et al. Development and validation of a deep learning system for diabetic retinopathy and related eye diseases using retinal images from multiethnic populations with diabetes. Jama. 2017;318(22):2211–23.
Topol EJ. High-performance medicine: the convergence of human and artificial intelligence. Nat Med. 2019;25(1):44–56.
Yasaka K, Akai H, Kunimatsu A, Abe O, Kiryu S. Liver fibrosis: deep convolutional neural network for staging by using Gadoxetic acid-enhanced hepatobiliary phase MR images. Radiology. 2018;287(1):146–55.
Chahal D, Byrne MF. A primer on artificial intelligence and its application to endoscopy. Gastrointest Endosc. 2020;92(4):813–20.e4.
Le Berre C, Sandborn WJ, Aridhi S, Devignes MD, Fournier L, Smaïl-Tabbone M, et al. Application of artificial intelligence to gastroenterology and Hepatology. Gastroenterology. 2020;158(1):76–94.e2.
Min JK, Kwak MS, Cha JM. Overview of deep learning in gastrointestinal endoscopy. Gut Liver. 2019;13(4):388–93.
Sinonquel P, Eelbode T, Bossuyt P, Maes F, Bisschops R. Artificial intelligence and its impact on quality improvement in upper and lower gastrointestinal endoscopy. Dig Endosc. 2021;33(2):242–53.
Suzuki H, Yoshitaka T, Yoshio T, Tada T. Artificial intelligence for cancer detection of the upper gastrointestinal tract. Dig Endosc. 2021;33(2):254–62.
Li J, Zhu Y, Dong Z, He X, Xu M, Liu J, et al. Development and validation of a feature extraction-based logical anthropomorphic diagnostic system for early gastric cancer: a case-control study. EClinicalMedicine. 2022;46:101366.
Takenaka K, Ohtsuka K, Fujii T, Oshima S, Okamoto R, Watanabe M. Deep neural network accurately predicts prognosis of ulcerative colitis using endoscopic images. Gastroenterology. 2021;160(6):2175–7.e3.
Wu L, Zhang J, Zhou W, An P, Shen L, Liu J, et al. Randomised controlled trial of WISENSE, a real-time quality improving system for monitoring blind spots during esophagogastroduodenoscopy. Gut. 2019;68(12):2161–9.
Zhou J, Wu L, Wan X, Shen L, Liu J, Zhang J, et al. A novel artificial intelligence system for the assessment of bowel preparation (with video). Gastrointest Endosc. 2020;91(2):428–35.e2.
Aoki T, Yamada A, Aoyama K, Saito H, Tsuboi A, Nakada A, et al. Automatic detection of erosions and ulcerations in wireless capsule endoscopy images based on a deep convolutional neural network. Gastrointest Endosc. 2019;89(2):357–63.e2.
Aoki T, Yamada A, Kato Y, Saito H, Tsuboi A, Nakada A, et al. Automatic detection of blood content in capsule endoscopy images based on a deep convolutional neural network. J Gastroenterol Hepatol. 2020;35(7):1196–200.
Aoki T, Yamada A, Kato Y, Saito H, Tsuboi A, Nakada A, et al. Automatic detection of various abnormalities in capsule endoscopy videos by a deep learning-based system: a multicenter study. Gastrointest Endosc. 2021;93(1):165–73.e1.
Ding Z, Shi H, Zhang H, Meng L, Fan M, Han C, et al. Gastroenterologist-level identification of small-bowel diseases and Normal variants by capsule endoscopy using a deep-learning model. Gastroenterology. 2019;157(4):1044–54.e5.
Fan S, Xu L, Fan Y, Wei K, Li L. Computer-aided detection of small intestinal ulcer and erosion in wireless capsule endoscopy images. Phys Med Biol. 2018;63(16):165001.
He J-Y, Wu X, Jiang Y-G, Peng Q, Jain R. Hookworm detection in wireless capsule endoscopy images with deep learning. IEEE Trans Image Process. 2018;27(5):2379–92.
Klang E, Barash Y, Margalit RY, Soffer S, Shimon O, Albshesh A, et al. Deep learning algorithms for automated detection of Crohn’s disease ulcers by video capsule endoscopy. Gastrointest Endosc. 2020;91(3):606–13.e2.
Noorda R, Nevárez A, Colomer A, Pons Beltrán V, Naranjo V. Automatic evaluation of degree of cleanliness in capsule endoscopy based on a novel CNN architecture. Sci Rep. 2020;10(1):17706.
Noya F, Alvarez-Gonzalez MA, Benitez R. Automated angiodysplasia detection from wireless capsule endoscopy. Annu Int Conf IEEE Eng Med Biol Soc. 2017;2017:3158–61.
Saito H, Aoki T, Aoyama K, Kato Y, Tsuboi A, Yamada A, et al. Automatic detection and classification of protruding lesions in wireless capsule endoscopy images based on a deep convolutional neural network. Gastrointest Endosc. 2020;92(1):144–51.e1.
Tsuboi A, Oka S, Aoyama K, Saito H, Aoki T, Yamada A, et al. Artificial intelligence using a convolutional neural network for automatic detection of small-bowel angioectasia in capsule endoscopy images. Dig Endosc. 2020;32(3):382–90.
Wang S, Xing Y, Zhang L, Gao H, Zhang H. A systematic evaluation and optimization of automatic detection of ulcers in wireless capsule endoscopy on a large dataset using deep convolutional neural networks. Phys Med Biol. 2019;64(23):235014.
Yuan Y, Meng MQ. Deep learning for polyp recognition in wireless capsule endoscopy images. Med Phys. 2017;44(4):1379–89.
Dutta A, Zisserman A. The VIA annotation software for images, audio and video. In: Proceedings of the 27th ACM International Conference on Multimedia (MM ’19), October 21–25, 2019, Nice, France. New York: ACM; 2019. p. 4. https://doi.org/10.1145/3343031.3350535.
Redmon J, Divvala S, Girshick R, Farhadi A. You only look once: Unified, real-time object detection. In: Proceedings of the IEEE conference on computer vision and pattern recognition; 2016. p. 779–88.
Bernal J, Tajkbaksh N, Sanchez FJ, Matuszewski BJ, Chen H, Yu L, et al. Comparative validation of polyp detection methods in video colonoscopy: results from the MICCAI 2015 endoscopic vision challenge. IEEE Trans Med Imaging. 2017;36(6):1231–49.
Pacal I, Karaboga D. A robust real-time deep learning based automatic polyp detection system. Comput Biol Med. 2021;134:104519.
Qadir HA, Shin Y, Solhusvik J, Bergsland J, Aabakken L, Balasingham I. Toward real-time polyp detection using fully CNNs for 2D Gaussian shapes prediction. Med Image Anal. 2021;68:101897.
He K, Zhang X, Ren S, Sun J. Deep residual learning for image recognition. In: Proceedings of the IEEE conference on computer vision and pattern recognition; 2016. p. 770–8.
Mascarenhas Saraiva M, Ribeiro T, Afonso J, Andrade P, Cardoso P, Ferreira J, et al. Deep Learning and Device-Assisted Enteroscopy: Automatic Detection of Gastrointestinal Angioectasia. Medicina. 2021;57(12):1378.
Martins M, Mascarenhas M, Afonso J, Ribeiro T, Cardoso P, Mendes F, et al. Deep-learning and device-assisted enteroscopy: automatic panendoscopic detection of ulcers and erosions. Medicina. 2023;59(1):172.
Cardoso P, Saraiva MM, Afonso J, Ribeiro T, Andrade P, Ferreira J, et al. Artificial intelligence and device-assisted Enteroscopy: automatic detection of enteric protruding lesions using a convolutional neural network. Clin Transl Gastroenterol. 2022;13(8):e00514.
Otani K, Nakada A, Kurose Y, Niikura R, Yamada A, Aoki T, et al. Automatic detection of different types of small-bowel lesions on capsule endoscopy images using a newly developed deep convolutional neural network. Endoscopy. 2020;52(9):786–91.
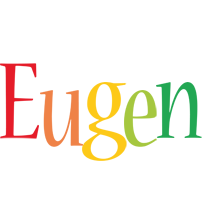
Eugen Boglaru is an AI aficionado covering the fascinating and rapidly advancing field of Artificial Intelligence. From machine learning breakthroughs to ethical considerations, Eugen provides readers with a deep dive into the world of AI, demystifying complex concepts and exploring the transformative impact of intelligent technologies.